Moving beyond averages: uncertainty and variability analysis in industrial ecology
Uncertainty and variability are inevitable in studying the environmental impacts of society’s use of resources – whether carbon emissions from producing steel and cement, or accumulation of heavy metals in municipal waste. Uncertainty arises partly because our knowledge about our natural and human systems is incomplete, and partly because of the impossibility of predicting the future. Variability refers to the variation of individuals: for example, even if we knew perfectly the average efficiency of cars, there is still a big range from the least to the most efficient.
This uncertainty and variability was the subject of a Special Session “Moving beyond averages: uncertainty and variability analysis in industrial ecology”, which I co-chaired together with Dr Jonathan Cullen, Leo Paoli and Dr David Laner, at the International Society for Industrial Ecology (ISIE) conference in Chicago in June 2017. This post will summarise the session, and collect the results of the discussion with the session participants.
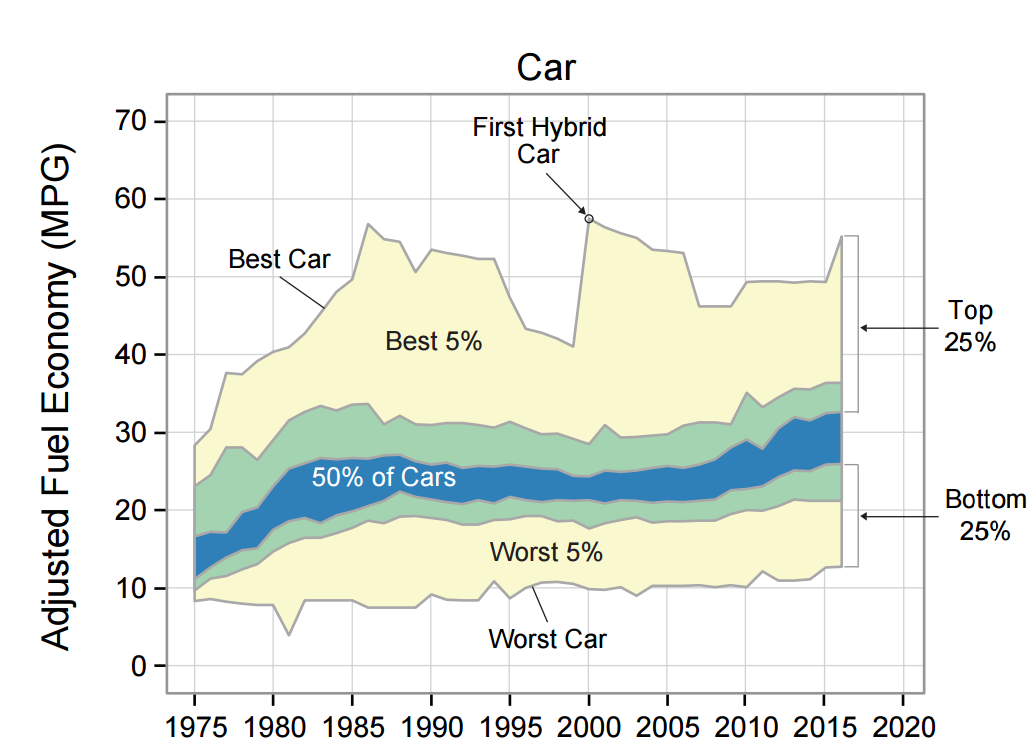
We structured the session around three stages of analysis with uncertainty: (1) assessing the quality of input data and characterising uncertainties; (2) inferring uncertain results given uncertain inputs; and (3) evaluating and communicating uncertain results. Jon introduced the session: you can see his slides here. For each part, David, Leo and I gave a short introduction, before opening up to discussion.
Data quality & characterising uncertainties
You can see David Laner’s slides here.
David started by discussing different types of uncertainty. One way of thinking about it is to distinguish aleatory variability, which is due to intrinsic randomness, from epistemic uncertainty, which is due to a lack of knowledge. Aleatory uncertainty cannot be reduced (e.g. outcome of tossing a coin), whereas epistemic uncertainty can be reduced by collecting more information (e.g. is there life on Mars?). Mostly here we were discussing epistemic uncertainty: we don’t have enough information to be sure about what’s happening now (such as exactly how much steel is used in different products), even though in principle we could find out, given enough time and effort.
Since then I’ve read a nice paper by David Spiegelhalter and Hauke Riesch which discusses these categories and others, and proposes a five-level framework for understanding different types of uncertainty:
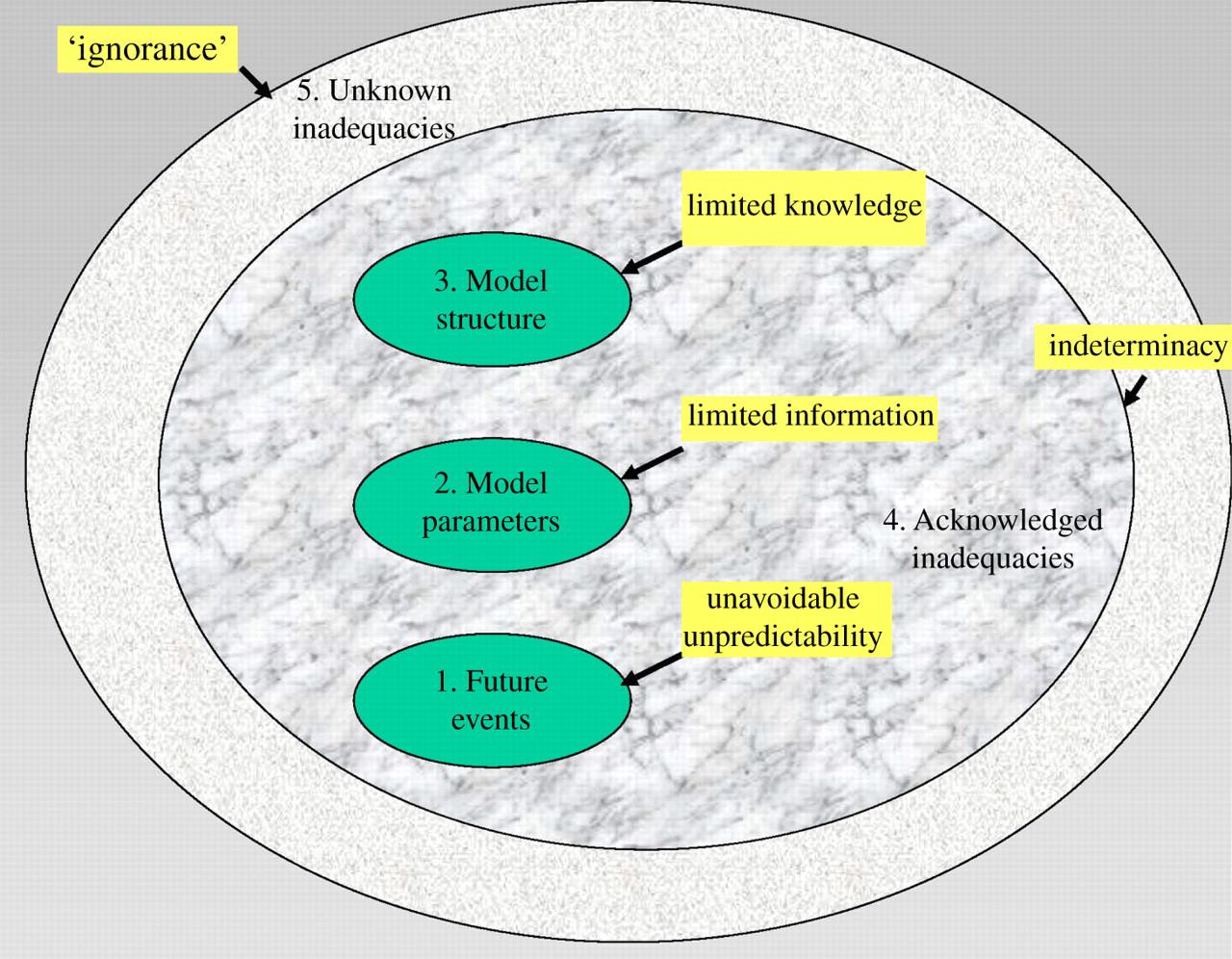
David described two steps to understanding uncertainty in the kind of data used in Industrial Ecology: firstly assessing the quality of the data, and then converting that qualitative assessment into a quantified uncertainty that can feed into modelling.
Data quality is “a measure of the reliability of data in the context of the application purpose” – it is not the same as measurement uncertainty. Imprecise data on the energy efficiency of buildings in the UK might be more appropriate than very complete and precise data on the energy efficiency of buildings in a very different climate such as Hawaii.
There are a number of methods that have been suggested for quantifying data quality using probability distributions or fuzzy sets, which David briefly reviewed.
Then we opened up to discussion. We tried to capture participants' responses using post-it notes for “key words”, “papers”, “tools” and “people” relevant to the three areas. At least from my experience of this part of the session, I don’t think this actually worked that well: while the discussion was good, the post-it notes failed to capture enough context to understand afterwards what their message was. Suggested papers are listed at the end of the post. Issues highlighted by the participants included:
- The choice of specific distribution functions for characterising uncertainty using probability distributions.
- The balance between complexity and efficiency
- That a basic level of information quality is needed before a detailed and systematic data quality assessment process is needed.
- Financial economics has experience of the subjectivity of the audience in interpreting the uncertainty.
Propagating uncertainty & inference
You can see my slides here.
Once you’ve characterised the uncertainty of the input data, this needs to be propagated through a model (in this context, usually some kind of MFA, LCA or IOA) to infer the uncertain estimate of relevant outputs.
I started by reviewing the methods used for doing this in these three types of modelling (although mostly I’m familiar with MFA). It’s striking that the most common method is “no method” – over half of studies in Esther Müller’s review of dynamic MFA of metals. I focused on what’s been called “parameter uncertainty”, at level 2 of David Spiegelhalter’s framework shown above. Mark Huijbregts also gives a broader view of sources of uncertainty and variability in LCA.
The aims of uncertainty propagation vary depending on the problem.
The basic question is: given uncertain data, how uncertain are our results?
Sometimes we have too much data, when we need to also ask: given conflicting sources, which should we choose?
More often, we have too little data, in which case uncertainty can act as a placeholder to give tentative results.
Based on these different situations, I mapped out the existing methods:
I picked out two areas of this map I find particularly interesting:
- On the left, when we have very little data. This is the subject of my paper Incremental Material Flow Analysis with Bayesian Inference.
- At the bottom right, comparing the effects of using different model structures with the same data.
Issues which came up in discussion here included:
- The importance of focusing on uncertainties that actually matter to the results. The first step should be sensitivity analysis: it’s relatively easy, and if the results are shown to be robust then there’s no need for more.
- Tools such as global sensitivity analysis, Monte Carlo simulation, Markov Chain Monte Carlo, analytical propagation, Machine Learning techniques, the successive principle, and learning from Philosophy of Science to “embrace subjectivity”.
- The need to “take responsibility” to go in and look at the dataset – is it the right data?
- Most of these methods seem more concerned with uncertainty than variability: are different methods needed for variability?
Evaluating & communicating uncertain results
You can see Leo Paoli’s slides here.
Leo asked what we actually learn from uncertainty and variability analysis:
- At the most basic level, we learn about the uncertainty of our result: X ± Y.
- Given this we can compare uncertain results: which is more reliable?
- We can talk about uncertainty of comparisons: how sure are we that A > B?
- Identifying the source of uncertainty can guide further data collection to reduce it.
- Extreme cases tell us about the probability of reaching critical values and benchmarks.
Leo then presented some examples of different ways uncertainty has been communicated and how they address one or more of these outcomes: see his slides for details.
Tools for communicating uncertainty identified in the discussion included:
- Discernibility analysis (LCA)
- Sankey diagrams with colour to show uncertainty (two examples).
- Box and whisker plots, uncertainty cloud plots.
- Bringing in stakeholders at different points in the analysis (not just the end).
- Simplicity – tailoring to the expertise of the audience.
Suggested reading from session participants
Here are the papers suggested by participants in the workshop. My apologies if I missed something – I couldn’t read all the post-it notes – please let me know if you have anything to add!
J.R. Gregory, A. Noshadravan, E.A. Olivetti, and R.E. Kirchain. A Methodology for Robust Comparative Life Cycle Assessments Incorporating Uncertainty. Environmental Science & Technology (2016).
M.G. Morgan and M. Henrion. Uncertainty: A guide to dealing with uncertainty in quantitative risk and policy analysis. Cambridge University Press (1990).
M.A. Mendoza Beltran, J. Guinée et al. Uncertainty in LCA – what can be concluded?. Environmental Science and Technology (accepted 2017).
K. Zhao, T.S. Ng, H.W. Kua & M. Tang. Modeling environmental impacts and risk under data uncertainties. IISE Transactions (2017).
Applications:
D. Cooper. Evaluating emerging technologies. Journal of Industrial Ecology (under revision 2017).
D. Cooper, K.E. Rossie, T.G. Gutowski. The energy requirements and environmental impacts of sheet metal forming: An analysis of five forming processes. Journal of MAterials Processing Technology (2017).
M. Rygaard, E. Arvin, P.J. Binning. The valuation of water quality: Effects of mixing different drinking water qualities. Water Research (2009).
Summary
Thank you to everyone who participated in the session, and to my co-chairs Jon, David and Leo. If you were there and think I missed something important, let me know!